About author
Aamir Ansari is a 25-year-old scientist from Nagpur. He did his bachelor’s in Chemical engineering from Visvesvaraya National Institute of Technology, Nagpur. He continued in the same discipline to persue his Master’s from Indian Institute of Science, Bangalore. Currently he is pursuing Ph.D. at the University of Minnesota, USA. He aspires to become a professor.
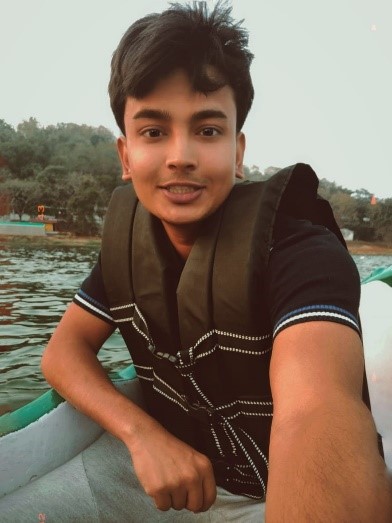
University of Minnesota, USA